Experiments
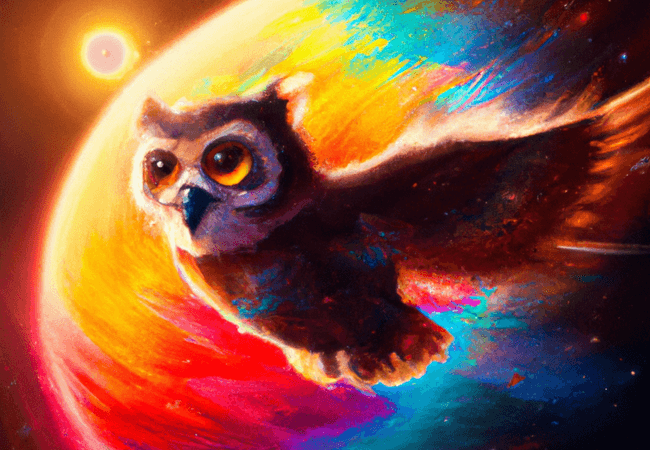
From Jupyter Notebook to DVC pipeline for reproducible ML experiments
In this guide we will take a Jupyter Notebook and use Papermill to turn it into a simple, one-stage DVC pipeline.
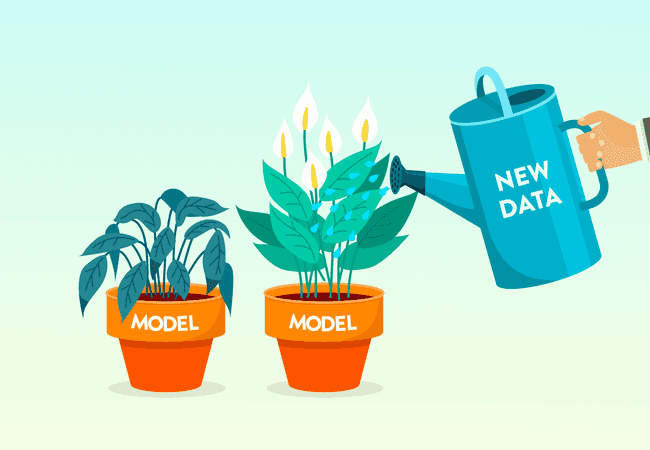
Preventing Stale Models in Production
We're going to look at how you can prevent stale models from remaining in production when the data starts to differ from the training data.
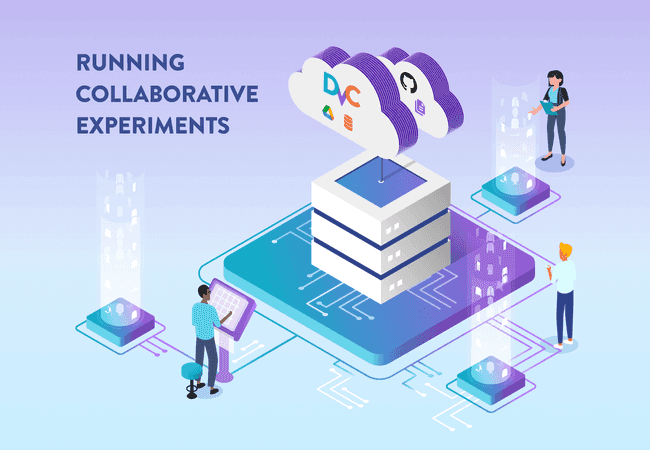
Running Collaborative Experiments
Sharing experiments with teammates can help you build models more efficiently.
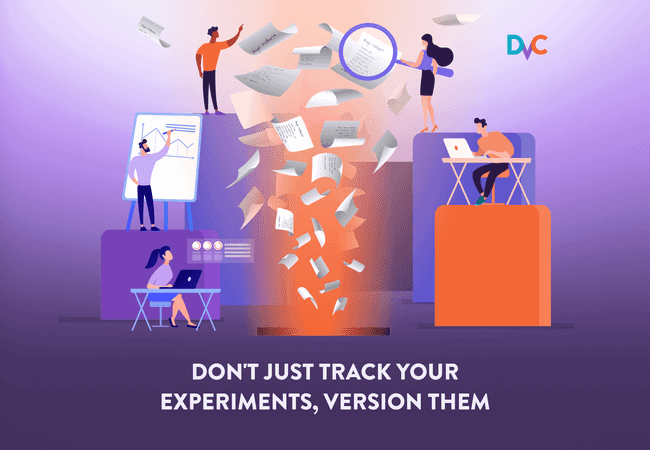
Don't Just Track Your ML Experiments, Version Them
ML experiment versioning brings together the benefits of traditional code versioning and modern day experiment tracking, super charging your ability to reproduce and iterate on your work.
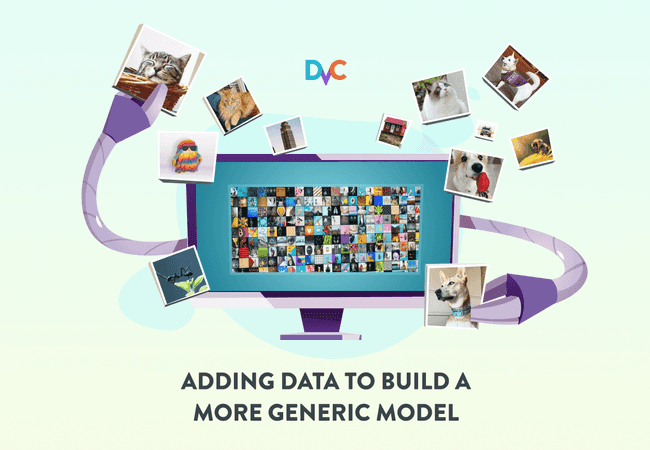
Adding Data to Build a More Generic Model
You can easily make changes to your dataset using DVC to handle data versioning. This will let you extend your models to handle more generic data.
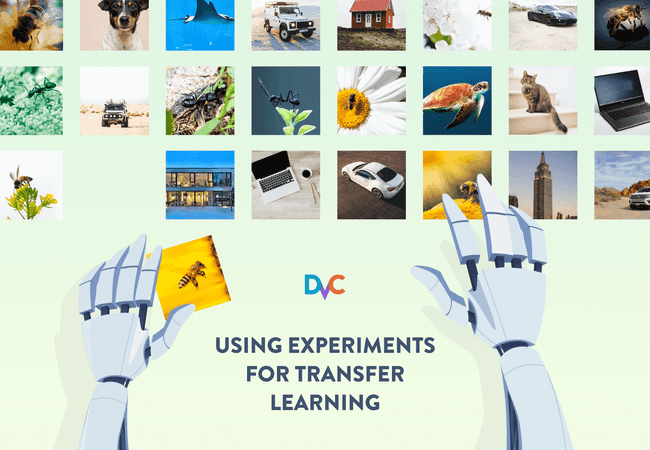
Using Experiments for Transfer Learning
You can work with pretrained models and fine-tune them with DVC experiments.
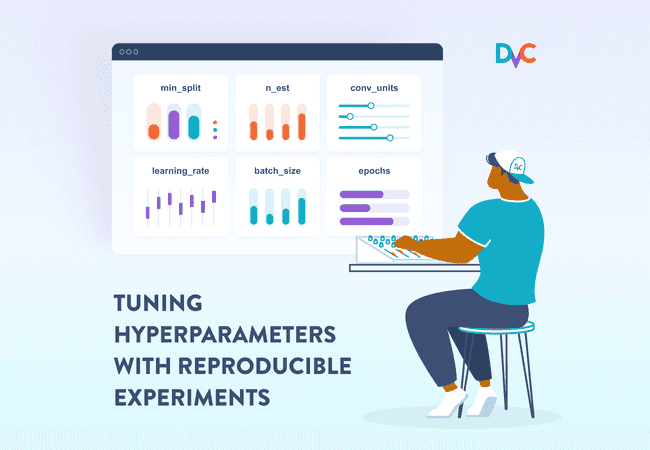
Tuning Hyperparameters with Reproducible Experiments
Using DVC, you'll be able to track the changes that give you an ideal model.
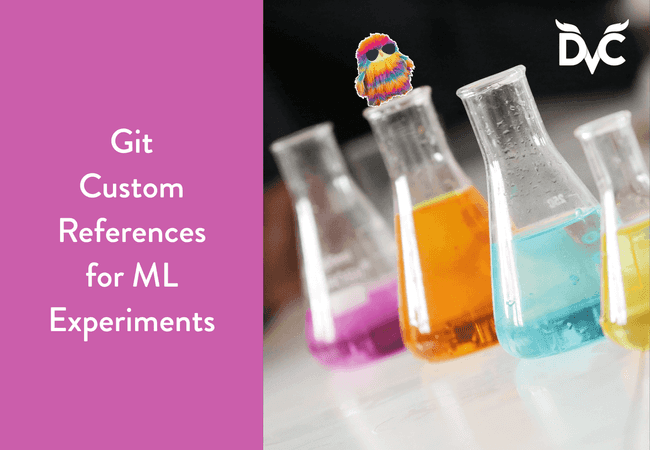
Git Custom References for ML Experiments
In DVC 2.0, we’ve introduced a new feature set aimed at simplifying the versioning of lightweight ML experiments. In this post, we’ll dive into how exactly these new experiments work.