MLOps
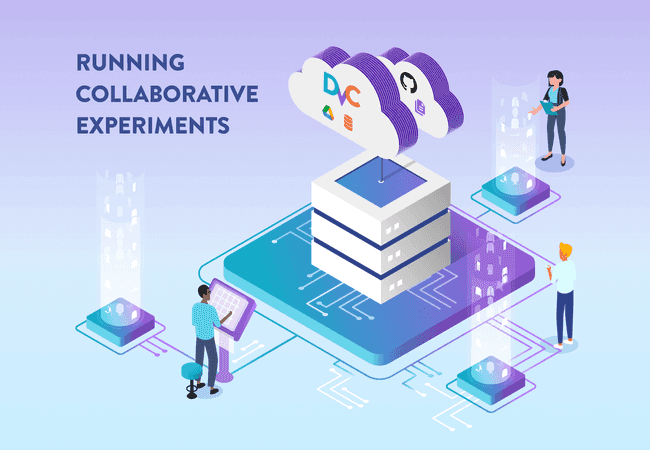
Running Collaborative Experiments
Sharing experiments with teammates can help you build models more efficiently.
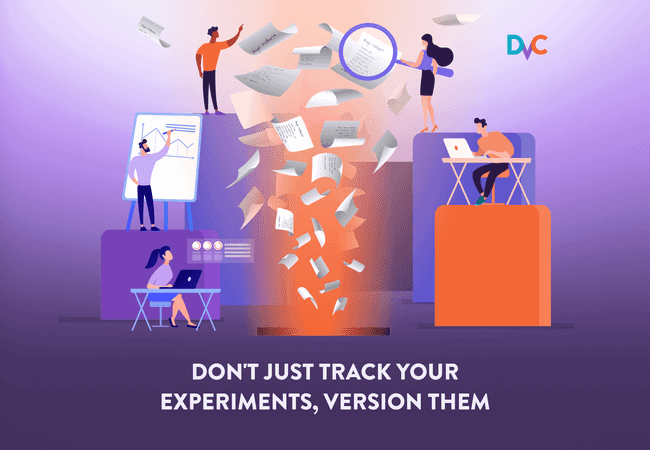
Don't Just Track Your ML Experiments, Version Them
ML experiment versioning brings together the benefits of traditional code versioning and modern day experiment tracking, super charging your ability to reproduce and iterate on your work.
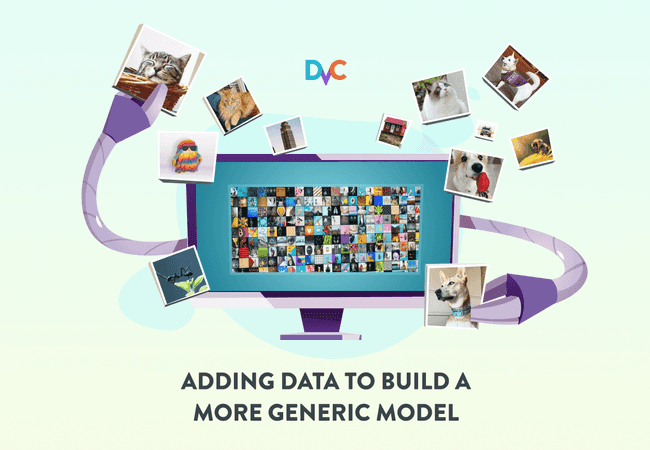
Adding Data to Build a More Generic Model
You can easily make changes to your dataset using DVC to handle data versioning. This will let you extend your models to handle more generic data.
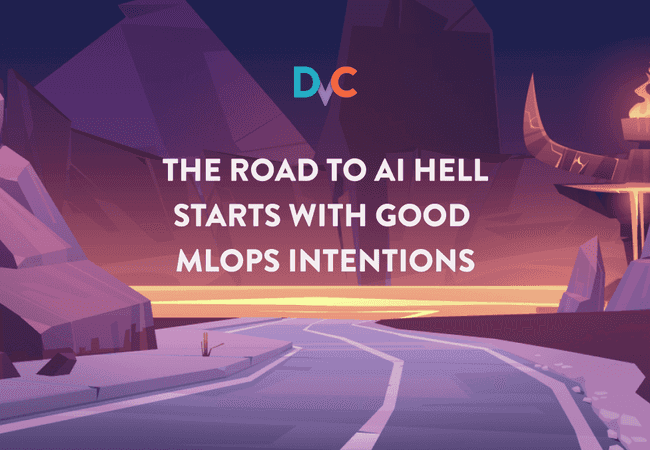
The Road to Hell Starts with Good MLOps Intentions
Why we believe extending best practices of software engineering to machine learning projects will streamline ML and AI development and keep all of us off the road to hell.
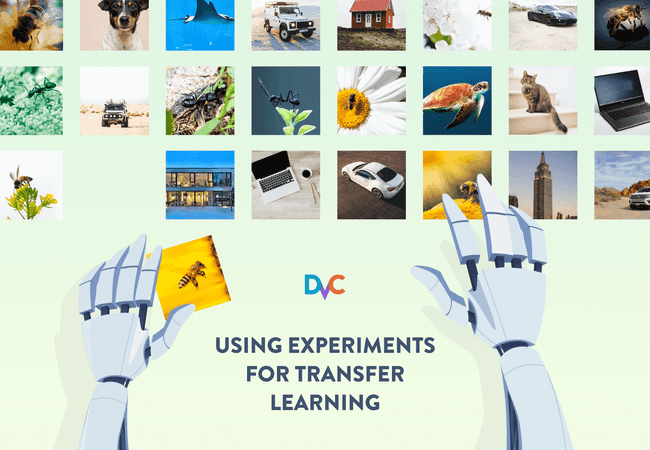
Using Experiments for Transfer Learning
You can work with pretrained models and fine-tune them with DVC experiments.
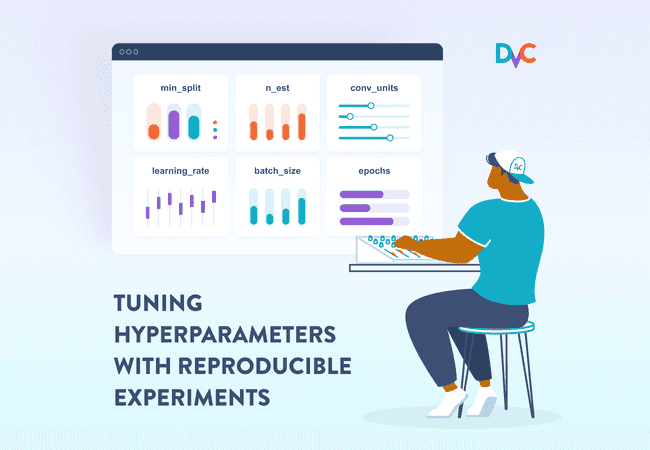
Tuning Hyperparameters with Reproducible Experiments
Using DVC, you'll be able to track the changes that give you an ideal model.
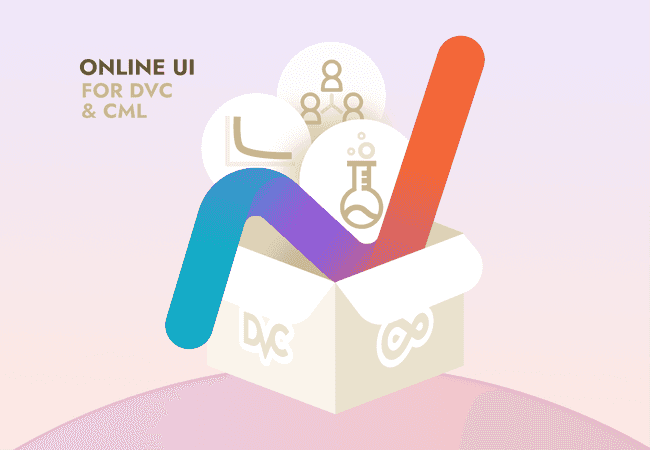
Introducing DVC Studio
🚀 We are excited to release DVC Studio, the online UI for DVC and CML. Use DVC Studio for ML versioning, visualization, teamwork and no-code automation on top of DVC and Git. Read all about the exciting features and watch videos to get started quickly.
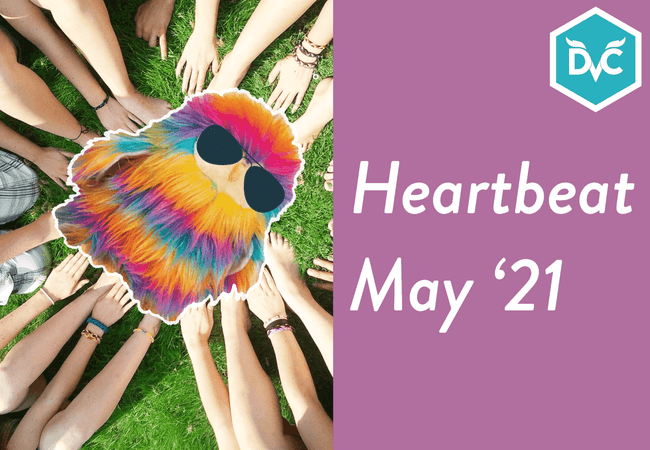
May ’21 Heartbeat
Monthly updates are here! We've hit 30 team members! MLOps learning opportunities, tutorials with integrations, conference videos, Discord server growth, and more!
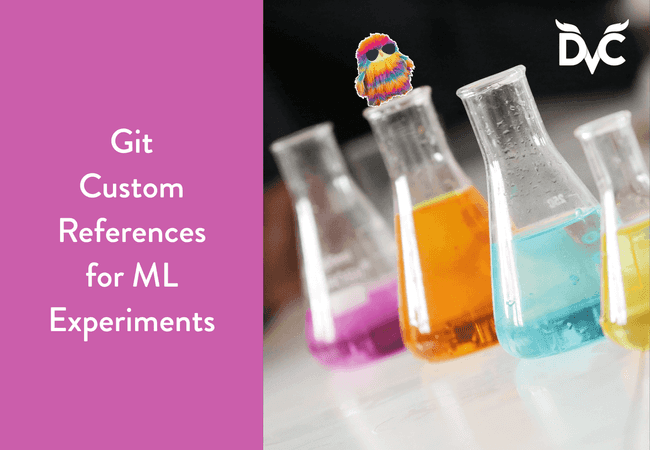
Git Custom References for ML Experiments
In DVC 2.0, we’ve introduced a new feature set aimed at simplifying the versioning of lightweight ML experiments. In this post, we’ll dive into how exactly these new experiments work.