Release
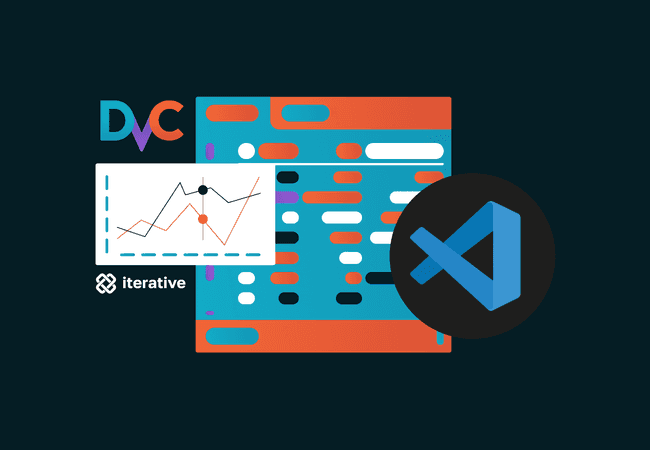
Turn Visual Studio Code into a machine learning experimentation platform with the DVC extension
Today we are releasing the DVC extension, which brings a full ML experimentation platform to Visual Studio Code.
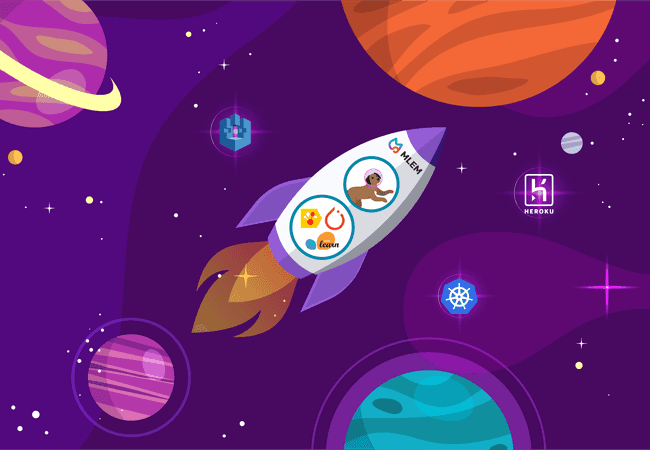
Productionize your models with MLEM in a Git-native way
Introducing MLEM - one tool to run your models anywhere.
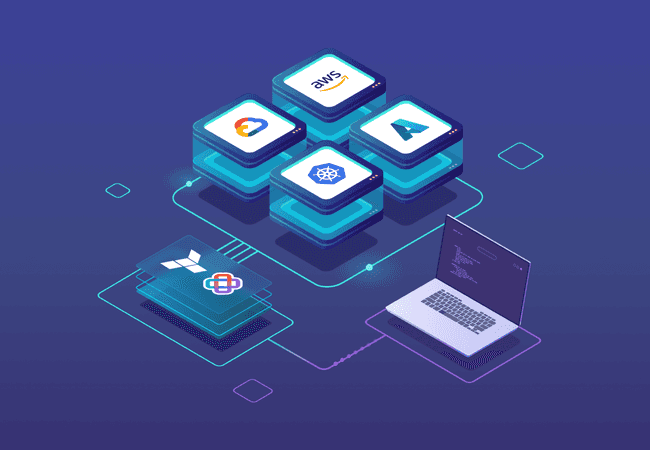
Machine Learning Workloads with Terraform Provider Iterative
Today we introduce painless resource orchestration for your machine learning projects in conjunction with HashiCorp Terraform.
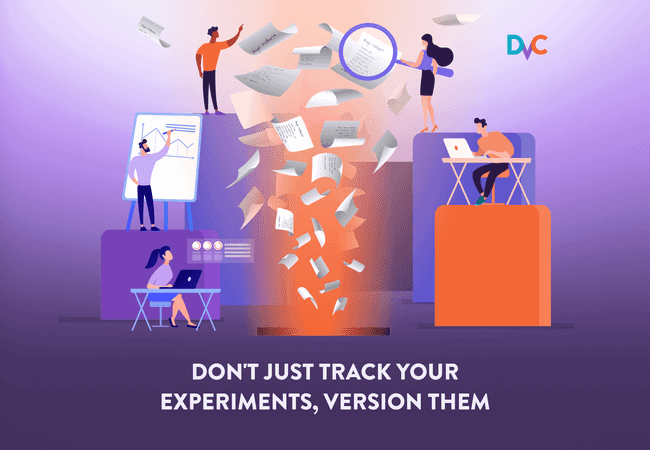
Don't Just Track Your ML Experiments, Version Them
ML experiment versioning brings together the benefits of traditional code versioning and modern day experiment tracking, super charging your ability to reproduce and iterate on your work.
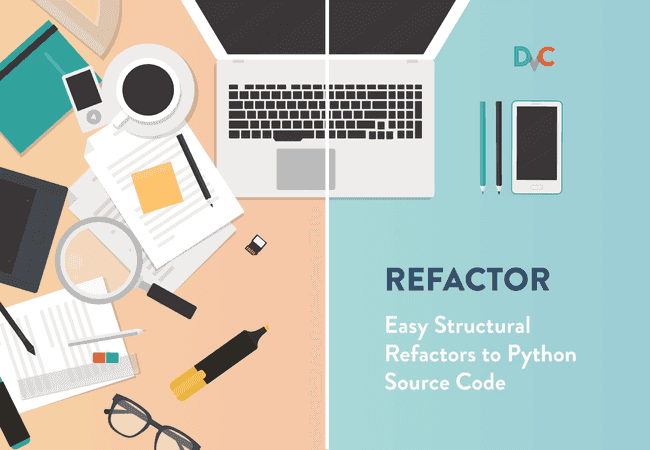
Easy Stuctural Refactors to Python Source Code
Simple, hassle-free, dependency-free, AST based source code refactoring toolkit.
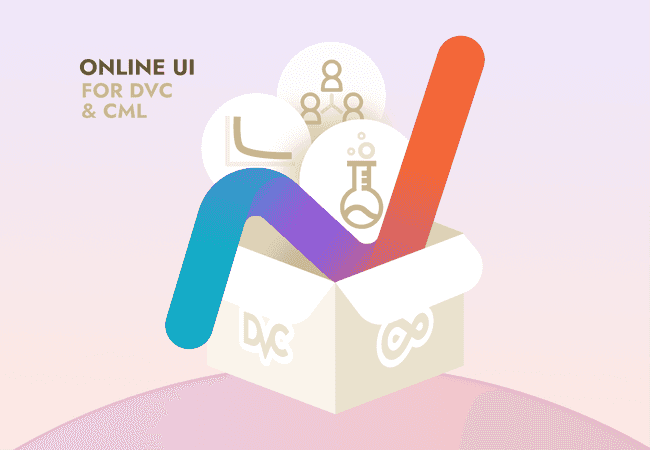
Introducing DVC Studio
🚀 We are excited to release DVC Studio, the online UI for DVC and CML. Use DVC Studio for ML versioning, visualization, teamwork and no-code automation on top of DVC and Git. Read all about the exciting features and watch videos to get started quickly.
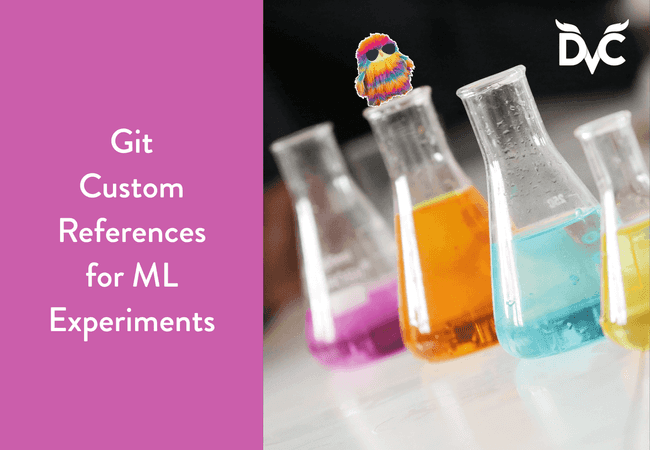
Git Custom References for ML Experiments
In DVC 2.0, we’ve introduced a new feature set aimed at simplifying the versioning of lightweight ML experiments. In this post, we’ll dive into how exactly these new experiments work.
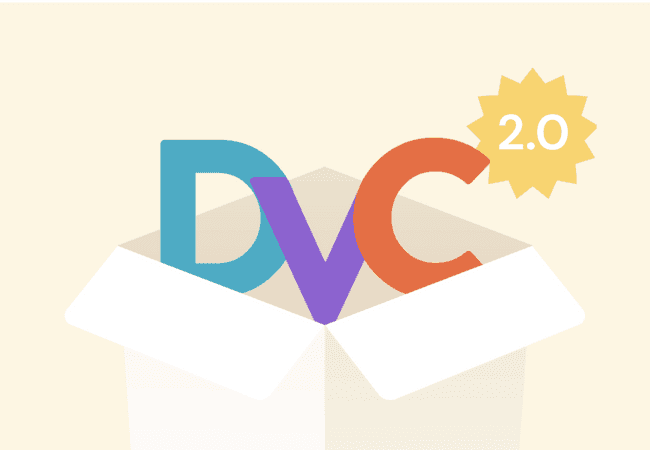
DVC 2.0 Release
Today is DVC 2.0 release day! Watch a video from DVC-team when we explain the new features and read more details in this blog post.
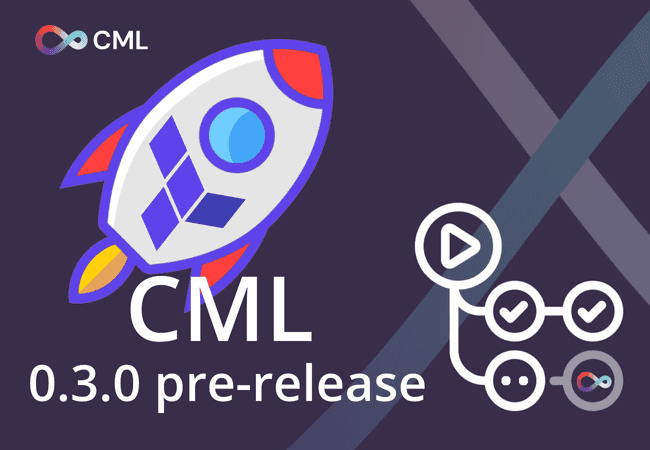
CML Pre-Release Notes: Automatically Train Models in the Cloud with CML 0.3.0
New features are here to make launching cloud compute for continuous integration workflows shorter, sweeter and easier than ever. Plus, a new GitHub Action to setup CML means more ways to use CML without our Docker container.