Tutorial
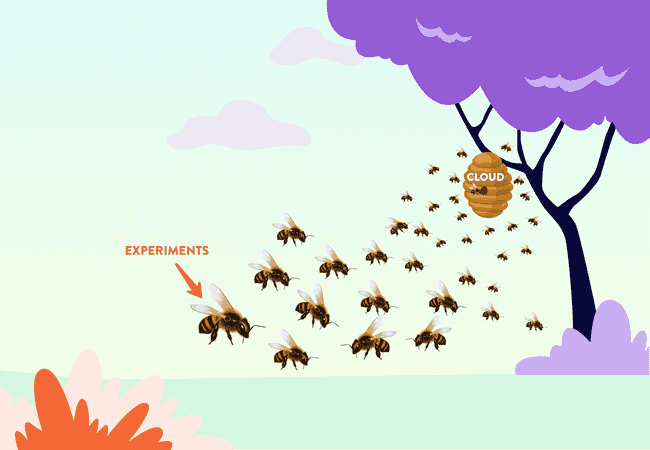
Moving Local Experiments to the Cloud with Terraform Provider Iterative (TPI)
Tutorial for easily moving a local ML experiment to a remote cloud machine with the help of Terraform Provider Iterative (TPI).
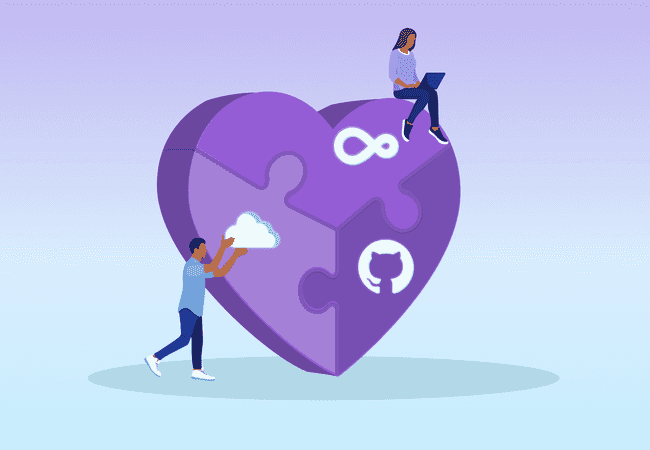
End-to-End Computer Vision API, Part 3: Remote Experiments & CI/CD For Machine Learning
In this final part, we will focus on leveraging cloud infrastructure with CML; enabling automatic reporting (graphs, images, reports and tables with performance metrics) for PRs; and the eventual deployment process.
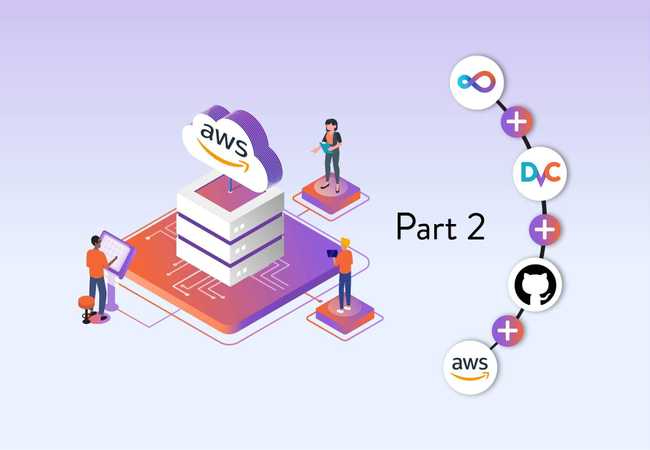
Training and saving models with CML on a dedicated AWS EC2 runner (part 2)
Use CML to automatically retrain a model on a provisioned AWS EC2 instance and export the model to a DVC remote storage on Google Drive.
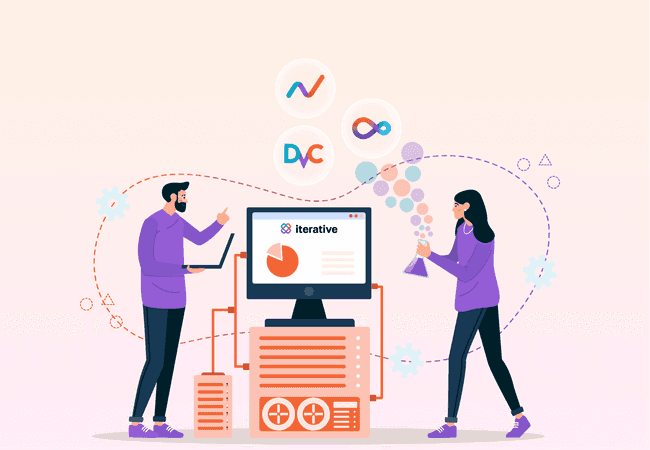
End-to-End Computer Vision API, Part 2: Local Experiments
In part 1, we talked about effective management and versioning of large datasets and the creation of reproducible ML pipelines.
Here we'll learn about experiment management: generation of many experiments by tweaking configurations and hyperparameters; comparison of experiments based on their performance metrics; and persistence of the most promising ones
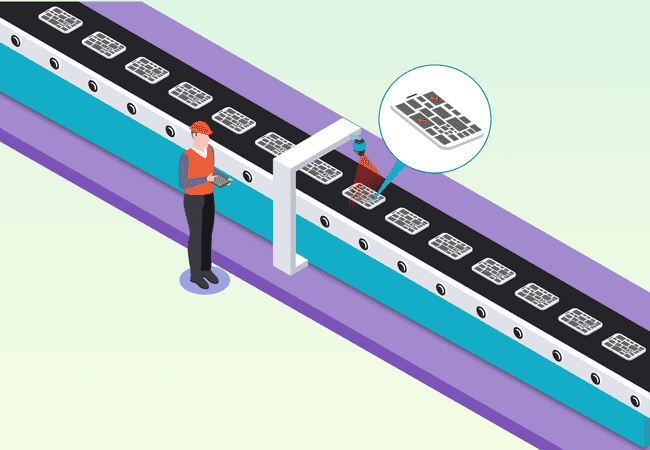
End-to-End Computer Vision API, Part 1: Data Versioning and ML Pipelines
In most cases, training a well-performing Computer Vision (CV) model is not the hardest part of building a Computer Vision-based system. The hardest parts are usually about incorporating this model into a maintainable application that runs in a production environment bringing value to the customers and our business.
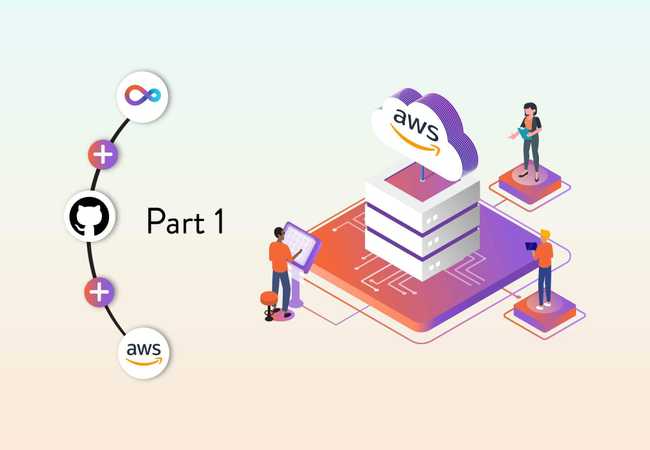
Training and saving models with CML on a self-hosted AWS EC2 runner (part 1)
In this guide we will show how you can use CML to automatically retrain a model and save its outputs to your Github repository using a provisioned AWS EC2 runner.
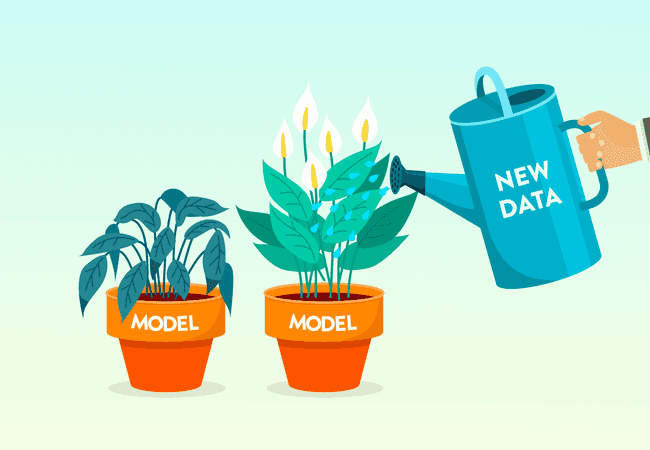
Preventing Stale Models in Production
We're going to look at how you can prevent stale models from remaining in production when the data starts to differ from the training data.
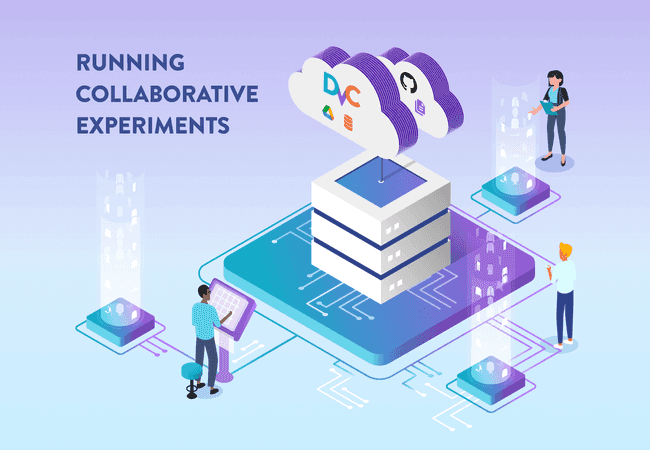
Running Collaborative Experiments
Sharing experiments with teammates can help you build models more efficiently.
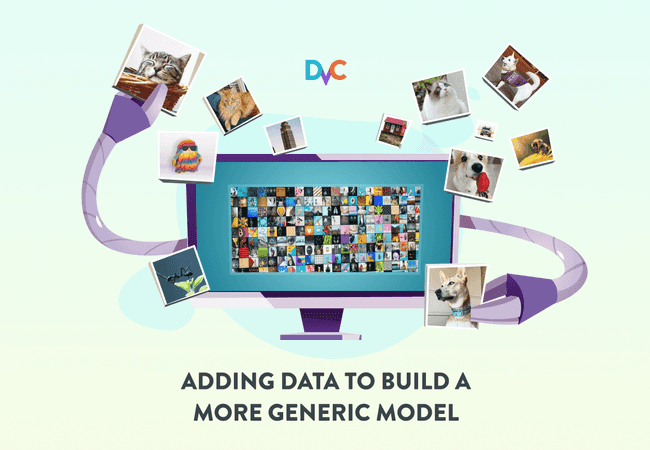
Adding Data to Build a More Generic Model
You can easily make changes to your dataset using DVC to handle data versioning. This will let you extend your models to handle more generic data.